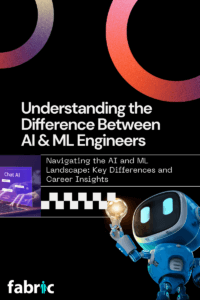
Understanding the Difference Between AI & ML Engineers
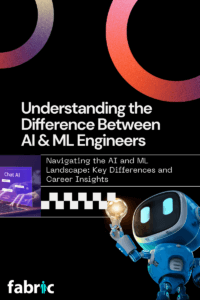
The rise of artificial intelligence (AI) has ignited a tech revolution, blurring the lines between science fiction and reality. But within this vast landscape, two crucial roles hold the keys to building these intelligent machines: AI engineers and machine learning engineers. While both titles might seem interchangeable, the nuances in their skill sets and focus areas create distinct career paths. This article delves into the specifics of these roles, highlighting their unique contributions to the tech industry.
Before diving into the specifics, let’s set the stage. AI is a broad umbrella term encompassing various techniques that enable machines to mimic human intelligence. Machine learning (ML), on the other hand, is a specific subset of AI that allows machines to learn from data without explicit programming.
Focus Areas
AI Engineer
AI engineers tackle a wide array of tasks that go beyond software development. They are involved in hardware integration, sensor fusion, and even mechanical design to create comprehensive intelligent systems. For example, they might work with cutting-edge robotics platforms, design AI-powered medical devices, or craft intelligent prosthetics.
Creativity and innovation are at the heart of an AI engineer’s work. They push the boundaries of AI, exploring novel approaches like reinforcement learning, generative models, and neuromorphic computing. This role often involves research, experimentation, and venturing into uncharted territory to unlock new possibilities for intelligent systems.
AI engineers also bridge the gap between AI and business. They collaborate with stakeholders to understand business needs and translate them into effective AI solutions. This involves cost-benefit analysis, ethical considerations, and ensuring these systems align with overall business goals.
Machine Learning Engineer
ML engineers are masters of data wrangling. They transform messy, incomplete, and ever-growing datasets into valuable insights through data cleaning, feature engineering, and anomaly detection. Their goal is to prepare data for model training.
While model accuracy is crucial, ML engineers understand it’s just one piece of the puzzle. They focus on factors like latency, explainability, fairness, and bias mitigation to ensure the models are not only accurate but also reliable, interpretable, and ethical in their predictions.
ML engineers are also DevOps champions. They build robust and scalable ML pipelines, integrate models into existing systems, and monitor their performance in real time. This role bridges the gap between development and operations, ensuring smooth deployment and continuous improvement of ML models.
Skillsets and Tools
AI Engineer
AI engineers often specialize in specific fields like robotics, natural language processing (NLP), or computer vision. As AI continues to develop more industries have sought AI tools leading for the need of engineers in specializations like finance, music, games, blockchain, agriculture, space, and much more. AI engineers delve deeper into the unique tools and techniques relevant to each domain, such as robotics simulation software, NLP libraries like spaCy or NLTK, or image processing frameworks like OpenCV.
Understanding hardware and infrastructure is crucial for AI engineers. They work with specialized hardware like GPUs, FPGAs, or neuromorphic chips to optimize performance and efficiency. Skills in continuous integration/continuous delivery (CI/CD) and machine learning operations (MLOps) are also valuable as AI systems become more complex.
Machine Learning Engineer
ML engineers handle large-scale data processing and model training using distributed computing and big data tools like Hadoop, Spark, or distributed TensorFlow. They are adept at experimentation and hyperparameter tuning, using tools for model selection and A/B testing to achieve optimal performance. Any company that needs to optimize data can benefit from an ML Engineer on staff.
Effective communication and visualization skills are essential for ML engineers. They must communicate findings and insights to non-technical audiences, utilizing strong data visualization skills and storytelling abilities.
Applications and Projects
AI Engineer
- Chatbot Development: AI engineers often work on creating sophisticated chatbots that engage in natural language conversations. This involves deep expertise in natural language processing, machine learning, and software engineering. These chatbots are used in customer service, healthcare, and other industries to provide seamless and intelligent user interactions.
- Self-Driving Cars: One of the most exciting applications of AI engineering is developing autonomous vehicles. This requires a combination of computer vision, robotics, and artificial intelligence to navigate complex environments safely. AI engineers work on algorithms that allow cars to recognize obstacles, interpret traffic signals, and make real-time driving decisions.
- Medical Devices: AI engineers also play a crucial role in the healthcare sector by designing AI-powered medical devices. These devices can assist in diagnostics, treatment planning, and patient monitoring. For example, AI can help create prosthetics that adapt to a patient’s movements or develop imaging systems that detect anomalies with higher accuracy than traditional methods.
The above are examples- skill requirements vary depending on each AI Engineer’s industry.
ML Engineer
- Recommendation Systems: Machine learning engineers excel in building recommendation systems that personalize user experiences. These systems analyze user behavior and preferences to suggest products, content, or services. Companies like Netflix, Amazon, and Spotify rely heavily on these systems to enhance customer satisfaction and engagement.
- Fraud Detection: In the financial sector, machine learning engineers develop models to detect fraudulent activities. By analyzing transaction patterns and identifying anomalies, these systems help protect businesses and consumers from financial losses. ML engineers need a strong grasp of data analysis and pattern recognition to build effective fraud detection algorithms.
- Customer Churn Prediction: Predicting customer churn is another vital application of machine learning. ML engineers create models that analyze customer behavior and predict which customers are likely to leave. This allows businesses to take proactive measures to retain valuable customers, improving overall customer loyalty and reducing turnover rates.
The above are examples- skill requirements vary depending on each ML Engineer’s industry.
The Synergy of AI and ML
AI and ML engineers often collaborate to create cutting-edge solutions that leverage the strengths of both fields. For instance, an AI engineer might develop an intelligent system that includes machine learning algorithms to improve its performance over time. Conversely, an ML engineer might utilize AI techniques to enhance their models’ capabilities.
Emerging Opportunities and Future Trends
As the boundaries between AI and ML continue to blur, new opportunities and hybrid roles are emerging. Engineers with a comprehensive understanding of both fields are particularly well-positioned to drive innovation and address complex challenges in various industries. Whether you’re passionate about advancing AI technology or mastering the intricacies of machine learning, the future holds exciting prospects for engineers in these dynamic and rapidly evolving fields.
Career Paths
Artificial Intelligence Engineer
AI engineers can specialize in niche markets like AI-powered security systems, AI generated art & music, or self-driving car technology. Opportunities exist in research and development roles within academic institutions and research labs. Entrepreneurial ventures also offer exciting possibilities, allowing AI engineers to develop innovative AI-powered products and services.
Machine Learning Engineer
ML engineers can work in data science and business intelligence teams, product development and optimization, or focus on model governance and ethical considerations. These roles involve analyzing complex datasets, building data-driven products, and ensuring the responsible development and use of AI.
Choosing the Right Path
Ultimately, the choice between AI and ML engineering boils down to individual passions and strengths. Whether you envision yourself building the next groundbreaking AI application or crafting powerful algorithms to unlock the secrets of data, both paths offer exciting opportunities to shape the future of AI.
As AI continues to evolve, the boundaries between these roles might blur further, leading to new specializations and hybrid positions. Regardless of the specific title, the focus should remain on fostering innovation and shaping the responsible development of artificial intelligence.
For more insights into AI and ML careers, follow our blog and stay updated on the latest trends and opportunities in the tech industry.
Check out our Best Tips on Hiring AI & ML Engineers